Artificial Intelligence (AI) has evolved into a dynamic field with diverse applications, driven by the various types of agents that power its capabilities. These agents, ranging from basic reflex mechanisms to advanced learning systems, play a pivotal role in how AI interacts with your users and solves problems.
According to a report by PwC, AI could contribute up to $15.7 trillion to the global economy by 2030, enhancing productivity and driving economic growth. This indicates the growing importance of AI agents in your workplace.
In this article, we will be exploring the seven different types of agents in AI. This short article will provide you with a list of the different types of AI agents and help you deploy the right agent for your business requirements.
What are Agents in AI?
In artificial intelligence, an agent is a system or program that perceives its surroundings, makes decisions, and takes actions to achieve specific goals. These agents work autonomously, meaning they do not require direct control by a human operator.
Agents can be categorized in various ways, depending on their traits. For example, they can be reactive (responding to immediate stimuli) or proactive (anticipating and planning for future needs). They can also operate in fixed or dynamic environments, where the environment may or may not change over time. Additionally, agents can be part of a single-agent system, where only one agent operates, or a multi-agent system, where multiple agents interact to achieve common goals.
The 7 different types of Agents in AI
Now that we have understood the meaning of AI agents, let’s explore the different types of Agents in detail.
Simple reflex agents
Simple reflex agents work by responding only to the current situation without considering past experiences or perceptions. These agents rely on a set of condition-action rules, which connect specific conditions to actions. If a condition is true, the agent performs the corresponding action; if not, it does nothing. This approach works well when the environment is fully observable, meaning the agent can see everything it needs to make decisions.
However, in environments where not everything is visible, these agents may get stuck in infinite loops. Randomizing their actions can sometimes help them avoid these loops.
Learning agents
A learning agent in AI is designed to improve its performance over time by learning from past experiences. It begins with basic knowledge but becomes better at making decisions and adapting through learning. A learning agent has four main components:
• Learning element
• Critic
• Performance element
• Problem generator
Model-based reflex agents
Model-based reflex agents operate by identifying a rule that fits the current situation. These agents are designed to handle partially observable environments by using a model of the world.
They maintain an internal state that gets updated with each new perception, relying on their perception history. This internal state serves as a representation of the unseen parts of the world, allowing the agent to make informed decisions even when some information is hidden.
Goal-based agents
Goal-based agents make decisions by evaluating how close they are to achieving their desired goal, which represents a specific outcome or situation. Each action they take is aimed at reducing the gap between their current state and the goal. This approach helps them choose the best option among several possibilities by focusing on actions that lead to the goal.
Their decision-making process is guided by explicit knowledge that can be updated, making them more adaptable and flexible. These agents typically rely on search and planning to determine their actions, and their behavior can be adjusted easily as goals change.
Utility-based agents
Utility-based agents are designed to make decisions based on maximizing their overall “happiness” or satisfaction. These agents are also known as problem solving agents. When faced with multiple options, these agents evaluate the best choice by considering a preference (utility) for each possible state.
Simply achieving a goal might not be enough; for example, an agent may prefer a quicker, safer, or cheaper way to reach a destination. Utility represents how satisfied the agent is with a particular state.
Due to the uncertainty in the environment, these agents choose actions that are expected to maximize their utility. A utility function helps by assigning a real number to each state, reflecting how “happy” the agent is in that situation.
Hierarchical agents
Hierarchical agents are structured in a way that higher-level agents manage and guide the actions of lower-level agents. The high-level agents set the goals and establish the rules, while the lower-level agents focus on completing specific tasks.
This system is particularly useful in complex environments where many tasks and sub-tasks need to be handled efficiently.
Multi-agent systems
Multi-agent systems (MAS) involve multiple agents that work together to reach a shared goal. These agents may need to coordinate their actions and communicate with each other to be successful.
In a MAS, each agent can be autonomous or semi-autonomous, meaning they can make their own decisions based on what they perceive in their environment. The agents collaborate by taking actions that move them closer to achieving the common objective.
Conclusion
The seven types of agents in AI showcase the breadth of possibilities in designing intelligent systems, each tailored to specific challenges and goals. From the simplicity of reflex agents to the adaptability of learning agents, these categories highlight the progression of AI towards greater efficiency and autonomy.
If you’re looking to build a customized AI agent to suit your unique business needs, Kenyt.AI is the ideal partner. Contact our sales team today to explore how we can help you bring your AI vision to life.
Frequently Asked Questions
The primary types of AI agents include Simple Reflex Agents, Model-Based Reflex Agents, Goal-Based Agents, Utility-Based Agents, Learning Agents, Multi-Agent Systems, and Autonomous Agents. Each has unique functionalities and applications.
Reflex Agents operate solely on the current state of the environment, while Goal-Based Agents consider future states to achieve specific objectives.
Learning Agents use feedback from their environment to adapt and enhance their performance, incorporating experiences to make better decisions in the future.
Multi-Agent Systems involve multiple agents working collaboratively or competitively to solve complex problems, making them essential for distributed AI applications like robotics and simulations.
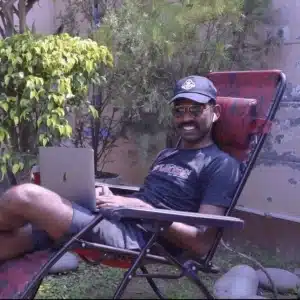
Aaron Jebin is an enthusiastic SAAS technical content writer interested in writing for new and existing technologies, platforms, and tools. With an experience of over 4 years in technical writing, he is keenly focused on developing articles to provide readers with complete solutions to the common problems that arise in the everyday workplace. His writing mostly focused on team building, work ethics, business analysis, project management, automation, AI, customer and employee engagement methodologies. He has an interest in baking cakes and making stained glass art. He is currently honing his drifting skills.