Knowledge-based agents in AI are built around the concept of service helping to perform tasks, simplifying our lives, and enhancing our capabilities. Today, these agents are organized in a hierarchy based on their specific functions and applications, each designed to serve different needs and purposes.
A recent survey found out that 81% of customers prefer using self-service options powered by AI agents before contacting a representative. This drastically huge number indicates the need for businesses to incorporate and deploy knowledge-based agents for your business applications.
In this article, we will be exploring what knowledge-based agents in AI are, their types, and practical applications in business use cases. By the end of this article, you will be able to understand the right type that is needed for your business.
Continue reading about other types of AI agents – What Are Problem Solving AI Agents In Artificial Intelligence
What are knowledge based agents in AI?
A knowledge-based agent in AI is essentially a computer program or system capable of sensing its environment through sensors and taking actions through actuators. It can make decisions and act on them autonomously, without human control. These agents are categorized based on their specific characteristics.
Similar to humans, enterprises, or even machines, AI is a rational agent. It evaluates past and present information to make decisions that lead to the most optimal and desirable outcomes. They not only simplify day-to-day activities for manual resources but also come in handy for interactive agents that have the ability to make thier own decisions.
What are the types of knowledge based agents?
Now that we have understood the meaning of knowledge-based agents, let’s now explore the different types of knowledge agents. This will further improve your understanding of such agents.
Simple Reflex Agents
A simple reflex agent makes decisions based solely on the current situation, without an internal model of the world. It follows pre-determined “condition-action” rules, reacting to its environment without considering future events. While easy to use, these agents struggle in complex, unpredictable environments.
Goal-Based Agents
Goal-based agents take decision-making further by considering the future effects of their actions. They have specific goals and evaluate whether their actions help achieve them. Whether it’s navigating a maze or playing a game, these agents plan and make complex decisions to achieve their objectives.
Utility-Based Agents
Utility-based agents also aim to reach a goal, but they focus on finding the optimal path. They assess different possible actions using a utility function, seeking to maximize performance according to a set measure. These agents are especially helpful when faced with multiple possible actions or outcomes.
Model-Based Reflex Agents
Model-based reflex agents have an internal world model, which helps them infer missing information and act based on both their current observation and the model. They can make more informed decisions by considering not only what they perceive but also what they know about the world.
Learning Agents
Learning agents improve their performance through experience. Starting with basic knowledge, they learn and adapt over time to optimize their actions. They are particularly useful in dynamic environments, such as personalizing advertising strategies by understanding customer preferences and adjusting accordingly.
Multi-Agent Systems (MAS)
Multi-agent systems consist of several agents working together, often to achieve shared, individual, or even conflicting goals. These agents can operate autonomously and interact with one another to perform tasks. MAS can be found in areas like autonomous driving, multi-robot factories, automated trading, and more.
Hierarchical Agents
Hierarchical agents are structured in levels, with high-level agents overseeing lower-level agents. The high-level agents provide goals and constraints, while the lower-level agents perform specific tasks. This structure is ideal for large systems where tasks need to be divided and managed at different levels.
Practical use-cases of knowledge based agents
Before wrapping up the article, let’s explore a few practical use-cases of knowledge-based agents in AI. This will help you understand the different applications of such agents in real-world business applications.
Intelligent Personal Assistants
AI-driven personal assistants like Siri, Alexa, and Google Assistant have become essential tools for millions. Once people started relying on them, their view of AI changed—it became a helpful companion, anticipating or following instructions.
As AI continues to evolve, it handles more tasks, from scheduling appointments and sending messages to setting reminders. These assistants are now a permanent part of daily life, making everyday activities more convenient.
Autonomous Machines
The dream of autonomous agents and robots performing physical tasks has long been a part of human imagination. Today, robotics is a reality. Robots now handle everything from household chores to complex machine assembly.
Home robots have gone from being sci-fi fantasies to affordable time-saving tools. In large warehouses, robots move and lift heavy objects, helping businesses like Amazon grow rapidly. Equipped with advanced sensors, these robots can safely interact with their environment, saving both time and money. Robotic agents use cameras, infrared sensors, and motors to perform tasks.
Competitive Gaming Bots
AI agents have made their mark in the world of gaming, evolving from simple responses to advanced competitors that can outperform human players. These gaming AI agents use deep learning and strategic decision-making, pushing the boundaries of research and development with every match. They compete against both humans and other AI, constantly improving their abilities and the gaming experience.
Systems for Fraud Detection
AI agents have become crucial in the fight against fraud, particularly in the finance sector. These agents analyze transaction patterns at incredible speeds to spot irregularities that may signal fraudulent activities or security breaches.
Banks and credit card companies rely on AI to monitor account activity and detect unusual transactions. With the ability to learn continuously and adapt, AI agents can catch new and evolving threats, making them highly effective at identifying fraud.
Conclusion
Knowledge-based AI agents mark the next innovative and interesting phase in humanity’s drive to scale up continuously. What started with shifting manual tasks to machines has now evolved into creating AI agents that possess abstract intelligence. These AI agents are truly remarkable, and as we continue to understand them more deeply, we can harness their potential to enhance both our productivity and efficiency.
Frequently Asked Questions
Knowledge-based agents in AI are systems that use a knowledge base to make decisions or solve problems. They rely on rules, facts, and reasoning techniques to interact with the environment and provide solutions.
The main types include rule-based agents, model-based agents, and reasoning agents. Rule-based agents use predefined rules, model-based agents build internal models of the world, and reasoning agents use logic to infer new information from known facts.
Knowledge-based agents are used in various applications, such as expert systems for medical diagnosis, recommendation engines in e-commerce, and decision support systems in business to automate decision-making processes and improve efficiency.
Knowledge-based agents enhance accuracy in decision-making by using structured data and rules. They can handle complex problems, provide insights, and support automation in industries like healthcare, finance, and customer service.
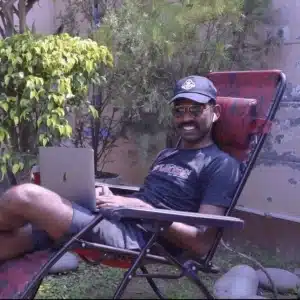
Aaron Jebin is an enthusiastic SAAS technical content writer interested in writing for new and existing technologies, platforms, and tools. With an experience of over 4 years in technical writing, he is keenly focused on developing articles to provide readers with complete solutions to the common problems that arise in the everyday workplace. His writing mostly focused on team building, work ethics, business analysis, project management, automation, AI, customer and employee engagement methodologies. He has an interest in baking cakes and making stained glass art. He is currently honing his drifting skills.