For years, AI has evolved from its roots in science, engineering, and mathematics into our everyday lives. Terms like “generative” and “predictive” hold significant meaning in this field. But why is it important to understand the difference between them? What do these concepts reveal, and how do they serve us in unique ways?
According to recent research, more than 52% of users say they use generative AI for everyday needs and trust the software to make informed decisions. Most of these users are Gen-Z and show the increasing demand and impact of this innovative technology on the market.
In this article, we will explore what is the difference between generative AI and predictive AI, so that you can understand the practical applications of both the technologies. This will help you deploy the right technology as per your requirement.
What is Generative AI?
Generative AI is designed to create original content by training on large amounts of data collected from the internet and other sources. It uses this data to identify patterns and produce new content that is similar to, but not identical to, the original. The outputs generated by generative AI aren’t direct copies or plagiarized but are inspired by the training data. When given a prompt, it generates “original” content like text, audio, images, software code, or even videos.
How Do Generative AI Models Work?
Generative AI models rely on neural networks to analyze patterns and structures in existing data, enabling them to create new, original content. This innovative technology works similar to the way a human brain would work.
A major advancement in generative AI is its ability to use different learning techniques, such as unsupervised or semi-supervised learning, during training. This approach allows organizations to efficiently utilize vast amounts of unlabeled data to develop foundation models. These models serve as a starting point for AI systems capable of performing a variety of tasks.
Examples of foundation models include GPT-3 and Stable Diffusion. GPT-3 powers applications like ChatGPT, which can generate essays or other written content from simple text prompts. Similarly, Stable Diffusion and a whole lot of other Generative AI platforms enable users to create photo-realistic images based on textual prompts.
What is predictive AI?
Predictive AI combines statistical analysis with machine learning to identify patterns in data and predict future outcomes. By analyzing historical data, it provides accurate forecasts about likely events, results, or trends. These models enhance the speed and accuracy of predictive analytics, commonly used in business forecasting—for example, predicting sales, estimating demand, personalizing customer experiences, or improving logistics. In doing so, predictive AI helps businesses make informed and strategic decisions about their next steps.
How Predictive AI Work?
Predictive AI uses Machine Learning (ML) algorithms to analyze historical data, understanding patterns, relationships, and trends. These models then use this information to predict future events. While humans are skilled, we often lack the computational power to identify these complex insights as effectively as advanced AI systems.
For example, predictive AI is highly valuable in industries like finance and marketing. It can forecast customer behavior, stock market trends, or product demand by processing large datasets and complex algorithms. This process helps in increasing the accuracy of insights and also significantly improves decision-making processes.
What is the difference between Generative AI and Predictive AI?
Here’s what you need to know about differentiating these two types of AI: Generative AI and predictive AI serve distinct purposes. Predictive AI focuses on solving problems by forecasting trends, helping businesses make smarter decisions. On the other hand, generative AI creates imaginative content, tackling challenges in more complex and creative areas.
To explore their differences further, let’s take a closer look with the table below.
Characteristics | Generative AI | Predictive AI |
---|---|---|
Purpose | Generates new content (e.g., images, text) by replicating human-like patterns. | Predicts future outcomes to deliver actionable business insights. |
Requirements | Requires a prompt or example to produce new content. | Analyzes historical data to generate predictions. |
Architecture model | Employs neural networks, GANs, and reinforcement learning techniques. | Utilizes statistical algorithms and machine learning models. |
Interpretation | Often lacks transparency in its decision-making process. | Depends on statistical data and requires human interpretation for insights. |
Advantages | Transforms content creation, automates workflows, boosts creativity, and supports industries like gaming and healthcare. | Provides accurate predictions, aids in informed decision-making, optimizes business processes, and improves customer experiences. |
Disadvantages | Requires significant resources, raises ethical concerns, may introduce bias, lacks transparency in decision-making, and carries the risk of AI “hallucinations.” | Relies on the quality of historical data, may struggle with unforeseen disruptions, and needs constant monitoring for accuracy. |
Applications | Applied in content generation, creative industries, healthcare, gaming, marketing, and advertising. | Commonly used in finance, marketing, e-commerce, supply chain management, and manufacturing. |
Conclusion
Both types of AI have an incredibly wide range of applications across industries, from healthcare to marketing, entertainment, manufacturing, and many more. However, their growing success and widespread use bring complex challenges, such as bias, privacy issues, job displacement, accuracy, and security concerns. With these opportunities come significant responsibilities, and leaders must fully engage with the challenges of AI when deciding to implement it.
At Kenyt.AI, we focus on providing systems and technologies that bring technology closer to the users. So whether it is a chatbot, virtual assistant, or a agent, you are in for making the right choice when you work with us. Contact our sales team today.
Frequently Asked Questions
Generative AI refers to artificial intelligence that creates new content, such as images, text, audio, or videos, based on patterns it has learned from training data. Examples include chatbots like ChatGPT and image generators like DALL·E.
Predictive AI focuses on analyzing historical data to forecast future outcomes or trends. It is commonly used in areas like sales forecasting, demand planning, and fraud detection.
Generative AI produces original outputs, while predictive AI makes forecasts or predictions based on patterns in existing data. Generative AI creates, whereas predictive AI anticipates.
Generative AI is widely used in content creation, marketing, and entertainment, while predictive AI is crucial for industries like finance, healthcare, and retail for making data-driven decisions.
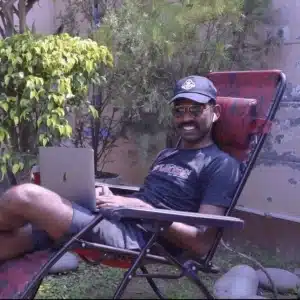
Aaron Jebin is an enthusiastic SAAS technical content writer interested in writing for new and existing technologies, platforms, and tools. With an experience of over 4 years in technical writing, he is keenly focused on developing articles to provide readers with complete solutions to the common problems that arise in the everyday workplace. His writing mostly focused on team building, work ethics, business analysis, project management, automation, AI, customer and employee engagement methodologies. He has an interest in baking cakes and making stained glass art. He is currently honing his drifting skills.